On impossible grammars and Large Language Models
The interest in Large Language Models (LLMs) has led to a resurgence of popular interest in cognitive science. One of the interesting questions that come up is whether these models understand language; that is, whether they have developed some form of cognitive ability. The results of models such as GPT4 and others is certainly extremely impressive and, as we have seen, the same approaches can be applied to the acquisition of a broad range of languages – including non-human languages such as the "language" of biology or chemistry. Theoretically, the same approach could be used to acquire a fictional language, such as Quenya (for readers of JRR Tolkein) and deliver the same degree of generative capability.
However, the way in which children acquire language is very different to the way in which artificial intelligence models are being taught language: children are not exposed to billions of pages of text in order to acquire competence in English-language grammer or expression.
They acquire high degrees of comprehension and expressiveness with relatively small amounts of data and, interestingly, at approximately the same pace regardless of whether they are learning English, Arabic, or Mandarin. If they learned using a purely statistical approach (similar to how Large Language Models work), one would expect that there would be random errors. However, this is not the case.
We also see cases where children have spontaneously invented languages – such as Nicaraguan Sign Language – without adult intervention or guidance.
Therefore, it raises the question of whether children have something inate that guides them to converge on a language – and whether this biological guide precedes the experience or exposure to language.
In 2016, Andrea Moro, a researcher in linguistics, published a fascinating book called Impossible Languages. Moro studied human languages and examined the syntax used across various language groups. Syntax is itself remarkable because it is what allows us, using a finite number of words, to create an infinite number of sentences and to express an infinite number of meanings.
In this study, he discovered that there are some syntactical rules that never appear in any human language. For example, there is no language that has a syntax such as "Anthony eats the no pear" (using a negative construction) to convey the meaning that I didn't eat a pear; and there is language that use an construction such as "Pear the eats Anthony". Moro characterised these as impossible grammers.
In his research, he conducted an experiment to determine whether there was something inate or biological guiding us towards the use of possible grammers which, if true, would explain the aforementioned phenomena of how childred acquire language.
The experiment was to take a number of test mono-lingual text subjects and monitor what parts of the brain are activated when performing different linguistic tasks. He created an artifical grammer consisting of easy but impossible rules – and taught these to the test subjects. Conversely, he taught another set of test subjects another language that conformed to the possible ruleset. The below table is an example:
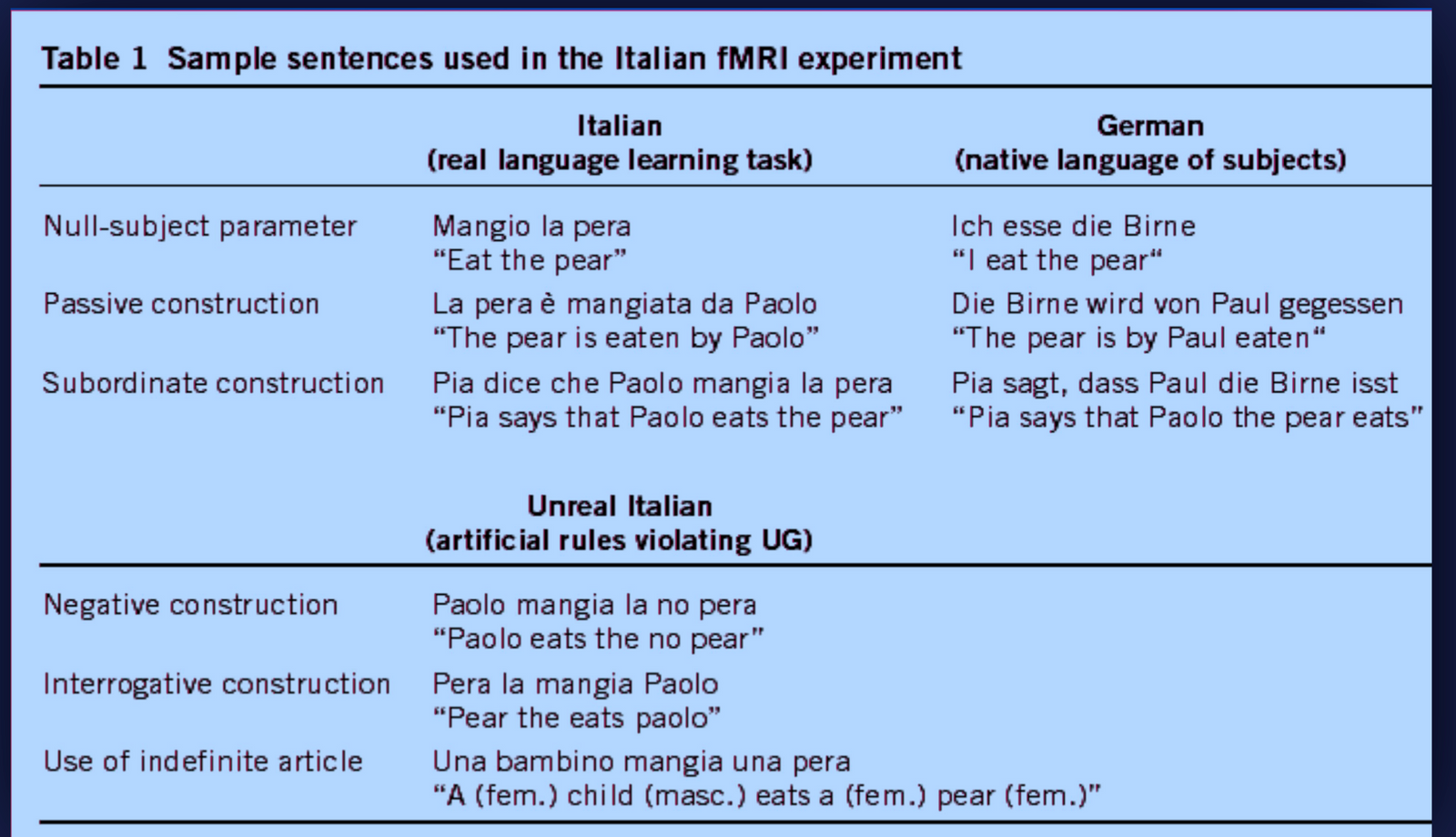
When the test subjects spoke their native German or Italian (using the correct Italian syntax), a part of the brain activated (known as Broca's area) that is used for language processing. When they spoke Italian using an impossible grammer, this activation didn't occur to the same degree but rather parts of the brain more associated with problem solving appear to have become active.
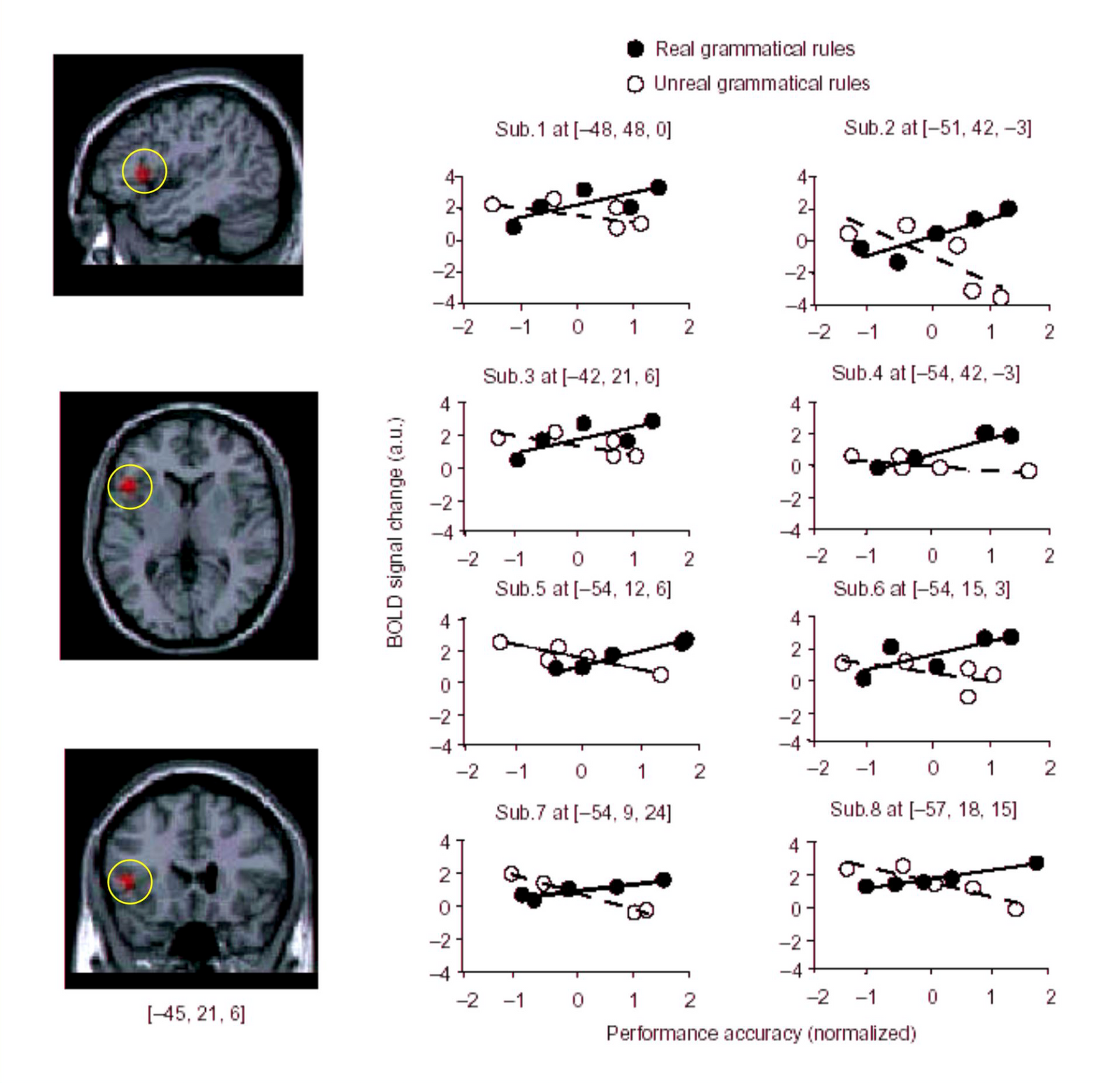
The implication of this is that, as Moro wrote, the "boundaries of Babel" are not cultural, learned or artificial; but rather it is imposed by the brain's structure or architecture. It does not matter what is our race, our culture, or our language: our brains seem, based on the research of Moro and others, to be somehow pre-programmed to converge on language acquisition or development within different hard-coded parameters (representing the range of possible grammers).
As we think then about building the artificial intelligence systems of the future, it is useful to consider that the fact that LLMs could acquire fictional languages that don't correspond to possible grammers with the same degree of efficiency as human langages points to a difference in how humans acquire language versus how neural networks do. It is, of course, possible that advanced capabilities may indeed be emergent in current approaches; but, perhaps, by understanding – and decoding – the architecture that seems to be inate in the human brain, we will develop new approaches that accelerate both the acquisition of language capabilities but, importantly, develop more sophisticated approaches to cognition that more closely resemble how humans acquire and utilise knowledge.
Anthony Butler Newsletter
Join the newsletter to receive the latest updates in your inbox.